SECURED InnoHub
SECURED InnoHub Concept
In SECURED, we aim to create and manage a Privacy-enhancing Hub (the SECURED InnoHub) that will provide tools, services, and overall support to external involved third parties of the healthcare domain, including researchers, Innovators or Health Data users as well as EU data Hubs across Europe, thus facilitating them to perform accurate data analytics in a distributed and private matter. The SECURED hub is also meant to promote collaboration among parties by acting as a one stop collaboration point for involved parties to share results and collaboratively shape/enhance their expertise through the Hub with others in a privacy-preserving matter. Given that the dominant health data analytics underlined technology is Machine Learning and Deep Learning intelligence, we focus our data analytics tools and services on enhancing the privacy of ML/DL solutions by offering a secure multiparty computation-capable toolbox that can operate in various modes under a SECURED Federation infrastructure. The end goal of the SECURED hub is to bring together providers and consumers of health data and offer them a trusted, secure and privacy-preserving environment to research, test their solutions and to collaborate.
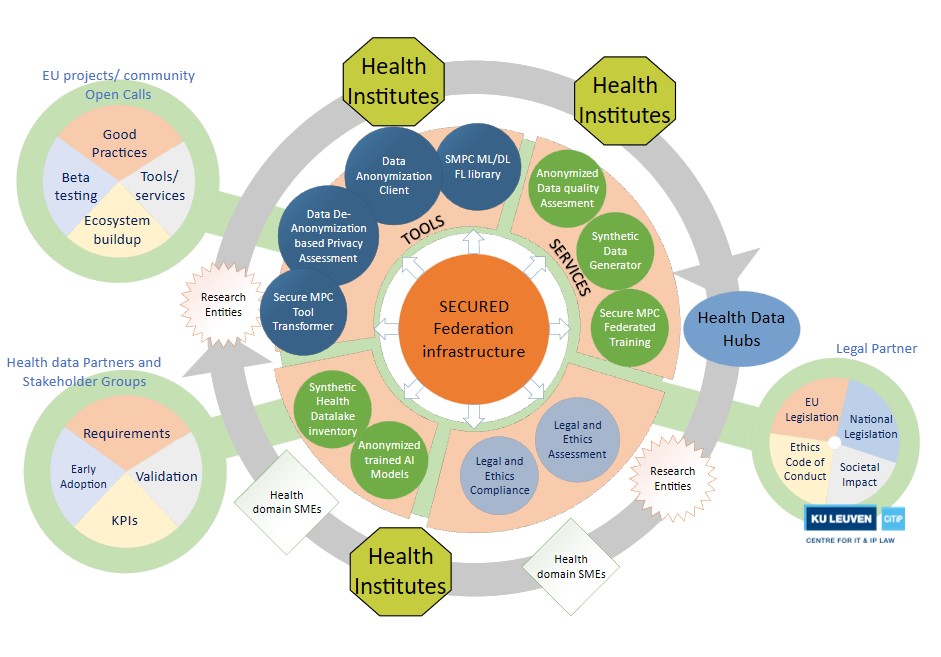