Federated Learning
in medical imaging
New Publication: Federated Learning for Breast Cancer Diagnosis in Real-World Clinical Settings
We are pleased to announce the publication of a new scientific article authored by partners of the SECURED and INCISIVE projects, highlighting a real-world case study in the application of Federated Learning (FL) for breast cancer diagnosis through mammography analysis.
Title: Towards generalizable Federated Learning in medical imaging: A real-world case study on mammography data
Published in: Computational and Structural Biotechnology Journal (Elsevier)
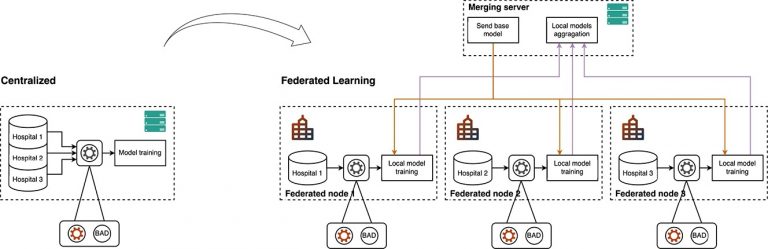
Summary
This study demonstrates how Federated Learning – a decentralized approach to machine learning – can be effectively applied in real clinical environments. In FL, sensitive medical data remains securely stored within each hospital while AI models are trained collaboratively across institutions. This method preserves patient privacy, ensures GDPR compliance, and allows for the development of robust, high-performance diagnostic models without transferring raw data between institutions.
The research was conducted by SECURE partners:
- Institute of Communication and Computer Systems (ICCS) of the National Technical University of Athens (NTUA)
- Barcelona Supercomputing Center (BSC-CNS)
In close collaboration with:
- Hellenic Cancer Society
- Aristotle University of Thessaloniki
- University of Novi Sad
Mammogram data from several hospitals in Greece and one in Serbia was used to train and validate an AI model for BIRADS classification, a standard used in breast cancer screening.
Key Contributions
Insight into Federated Learning challenges
A key takeaway from the study is that adapting centralized AI workflows to a federated architecture is not a trivial task. In real-world settings, researchers typically cannot access the raw data, which can obscure issues such as poor image quality, inconsistent annotation, or the presence of imaging artifacts (e.g. surgical clips, labels). This lack of visibility significantly affects performance.
The authors propose practical mitigation strategies, including:
- Deployment of data harmonization pipelines
- The appointment of Data Managers at each institution
- Standardization of preprocessing workflows
- Use of Federated Analytics to gain aggregate insights into the data
Introduction of the Breast Area Detection (BAD) tool
The study introduces a supervised deep learning module for automatic cleaning and standardization of mammography images, significantly improving data consistency across sites.
Validated real-world FL infrastructure
The Federated Learning framework developed in the study was tested in a live, multi-site clinical environment using hospital-based infrastructure. The model achieved performance levels comparable to classic centralized training, with improvements of up to 35% after harmonization interventions.
Broader Impact
The work directly supports the vision of SECURED to develop secure, anonymized, and scalable infrastructures for health data processing and AI model development across borders in Europe. The FL framework described in the paper forms part of the technical foundation for the EUCAIM project – Europe’s federated platform for cancer image analysis.
This case study represents a key step forward in demonstrating the feasibility, challenges, and best practices associated with deploying Federated Learning in sensitive healthcare contexts.
Funding Acknowledgement
This research was funded by the European Union’s Horizon 2020 research and innovation programme under the following grants:
- SECURED – Grant Agreement No. 101095717
- INCISIVE – Grant Agreement No. 952179
Follow our social media and subscribe to our newsletter to stay updated about the SECURED project and its ongoing contributions to secure health data processing and artificial intelligence.