Secured
Scaling Up Secure Processing, Anonymization And Generation Of Health Data For EU Cross Border Collaborative Research And Innovation
Objective 1
Provide Secure Multiparty Computation (SMPC) schemes for AI based health data analytics tools along with appropriate enhancements to allow them to scale up in realistic health domain scenarios.
Objective 2
Provide advanced Anonymization on Health datasets and AI models as well as assess the anonymity level using de-anonymization/re-identification techniques
Objective 3
Provide an adaptable, configurable, and versatile Synthetic-data-generation tools and services for health/medical synthetic data including synthetic images
Objective 4
Creation and Management of the SECURED Privacy-Preserving and Robust Federated-Learning Infrastructure (SECURED Federation Infrastructure) for scalability support of SECURED Health-data-related services and tools. Assure that the created FL models and the anonymized data used for AI training are unbiased.
Objective 5
Integration of the SECURED components and infrastructure. Create the SECURED Innohub that can offer a framework of tools and services as well as training and knowledge for a broad range of researchers, EU Data hubs, Innovators and end-users
Objective 6
Evaluate the SECURED solution and associated technologies in terms of legal and ethical aspects. Assess the legal status of cross-country usage of anonymized and synthetic datasets as well as AI models.
Objective 7
Validation and Demonstration with four use cases that involve Cross-Border EU health data hubs offering anonymized data and offering privacy preserving data analysis as well as support training and education.
Objective 8
Provide a viable dissemination, exploitation and business model of the SECURED solution that will build momentum and support the continuation of a SECURED privacy preserving collaborative health data ecosystem beyond the end of the project
SECURED Pilots
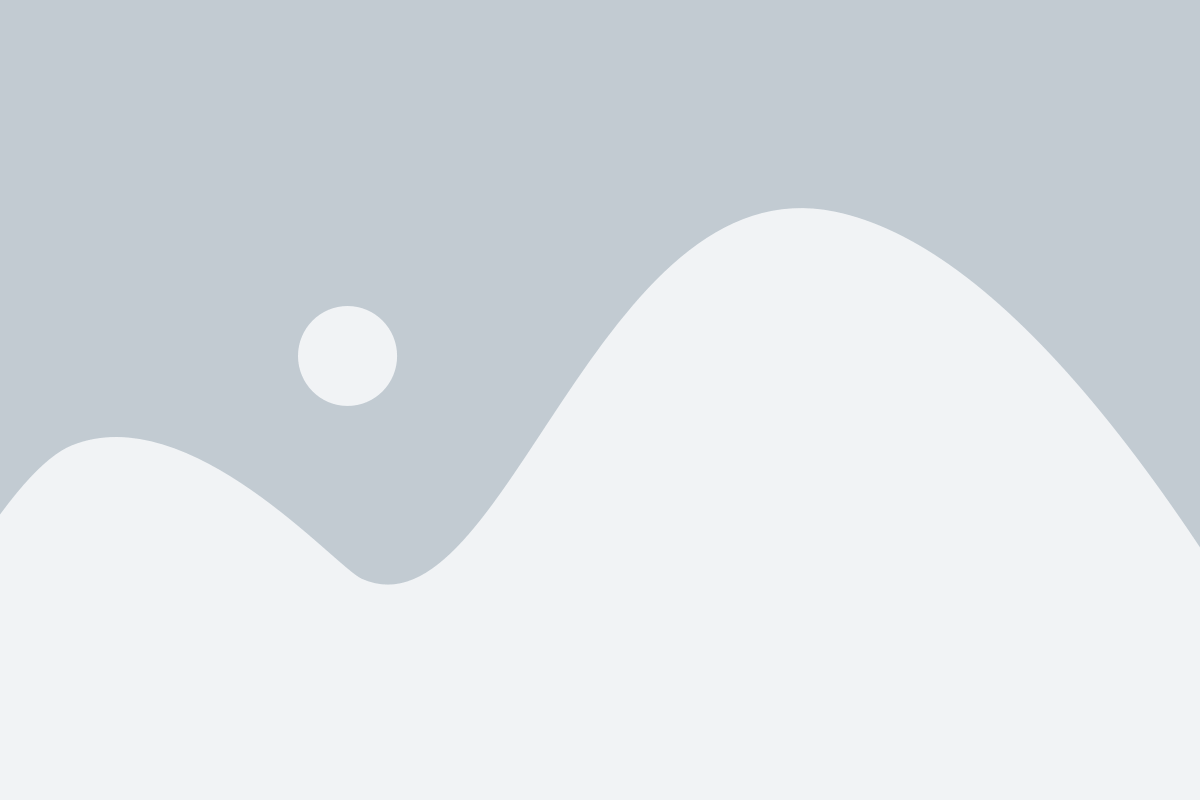
Pilot 1 (Erasmus Medical Center): Real-Time tumor classification
Using the SECURED architecture, this pilot expects using Ultrasound and Brain imaging to generate novel insights related to the correlation of anatomically separate but functionally connected brain regions, confirmations of previous connectivities and discovery of new ones.
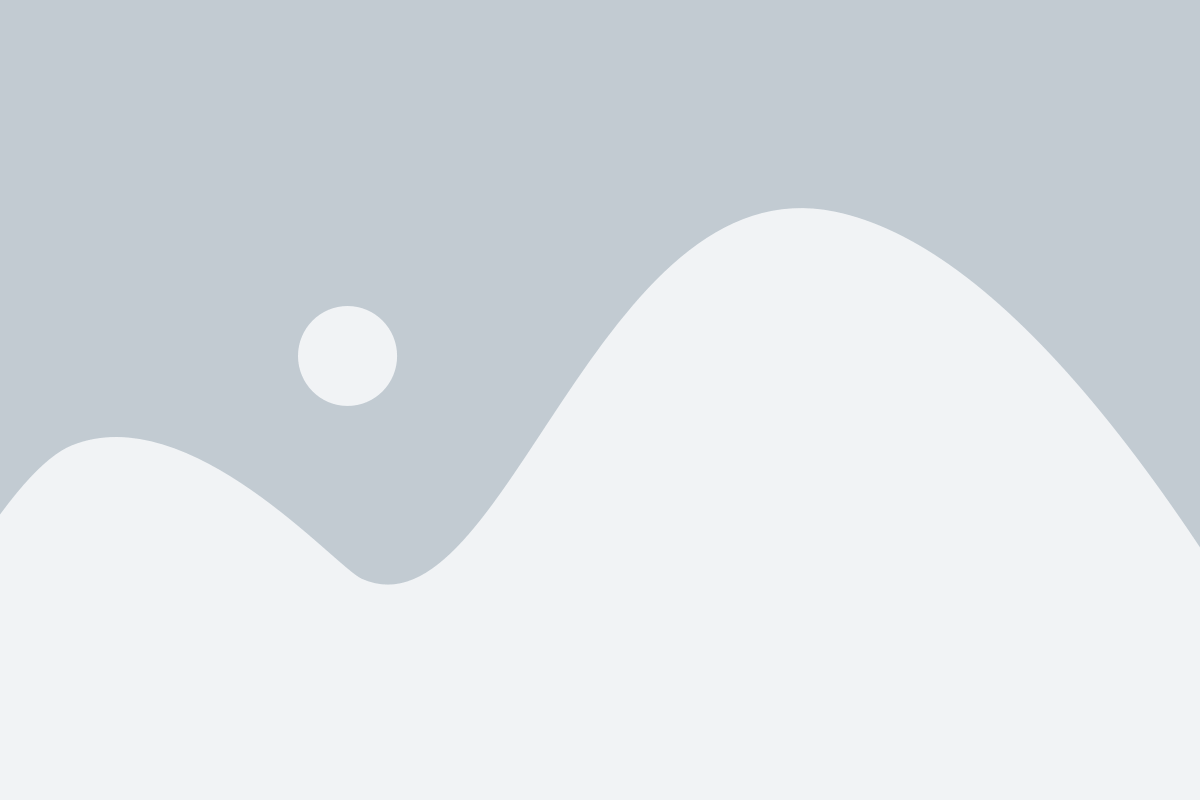
Pilot 2 (Paediatric Hospital Niño Jesús): Telemonitoring for children
Using the SECURED architecture, this pilot expects, in terms of prediction of bad evolution in oncology patients, the capability of using AI will foster the extension of telemedicine among paediatric patients, which can contribute significantly to meet goals 1, 3, 13, and 15 of 2030 Agenda for Sustainable Development.
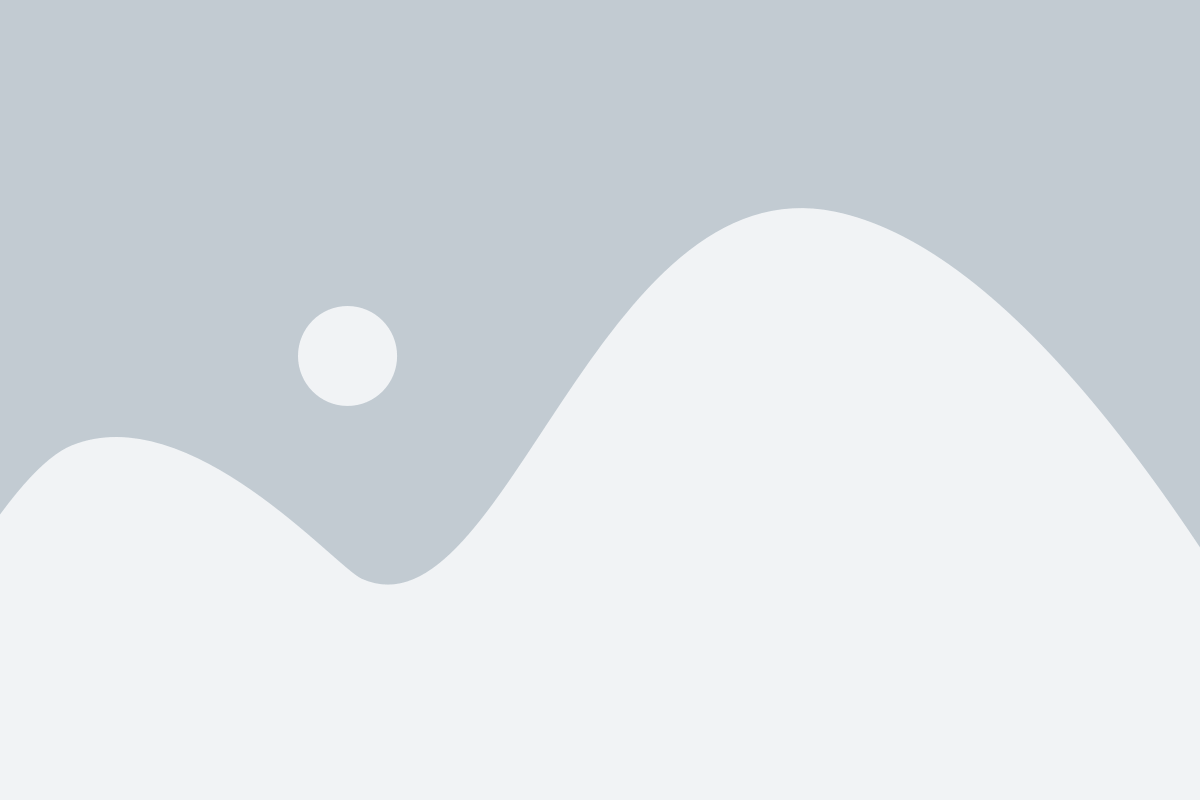
Pilot 3 (Semmelwies University): Synthetic-data generation for education
Using the SECURED architecture, this pilot expects to facilitate movement between countries and statistical data collection while protecting individual privacy, generate large amounts of synthetic data guaranteed to be GDPR compliant, educate doctors get a quick overview of millions of cases due to machine learning data synthesis instead of generalising from a single cases and finally doctors integrate machine learning tools into their daily practice.
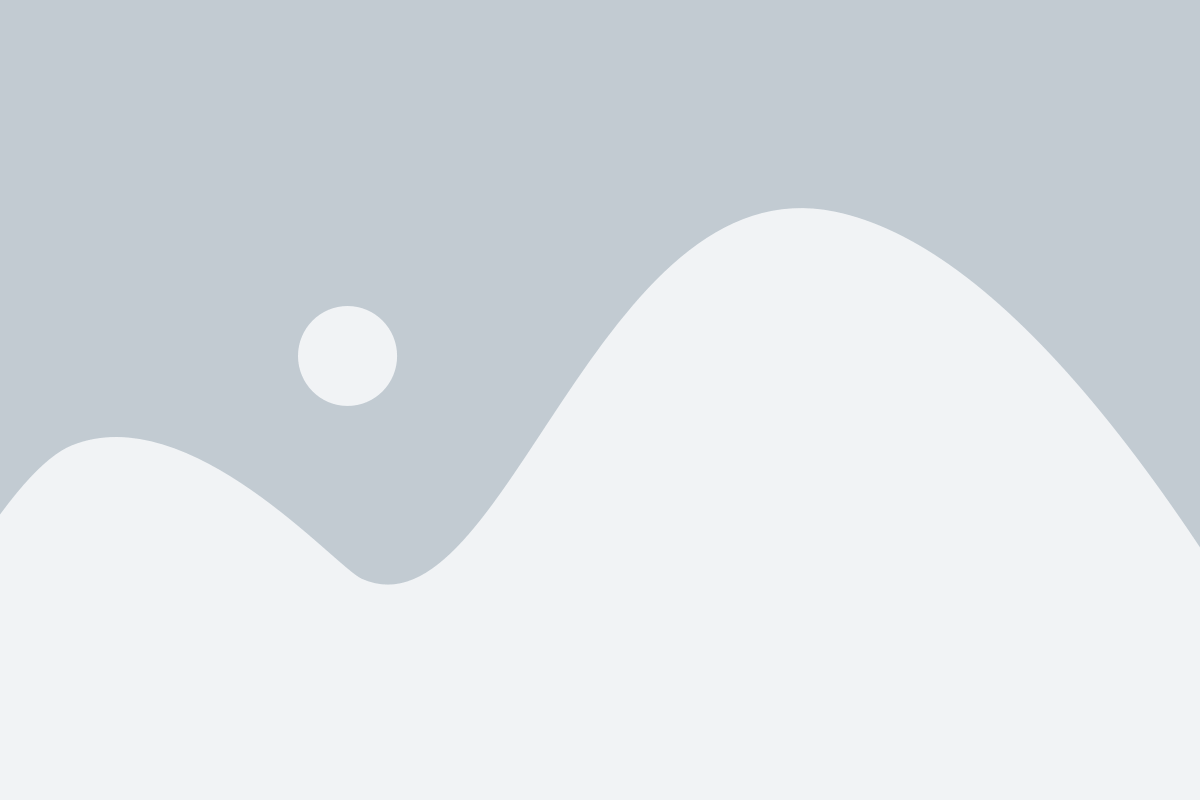
Pilot 4: (JCLRI) Access to Genomic Data
During this pilot, SECURED's federated learning system will allow researchers to train their models on all existing genetic data without the need of spending months preparing the paperwork to access it while, at the same time, preserving patient privacy, will transform the way genetics research is currently done.
Latest News and Events
Consortium Partners
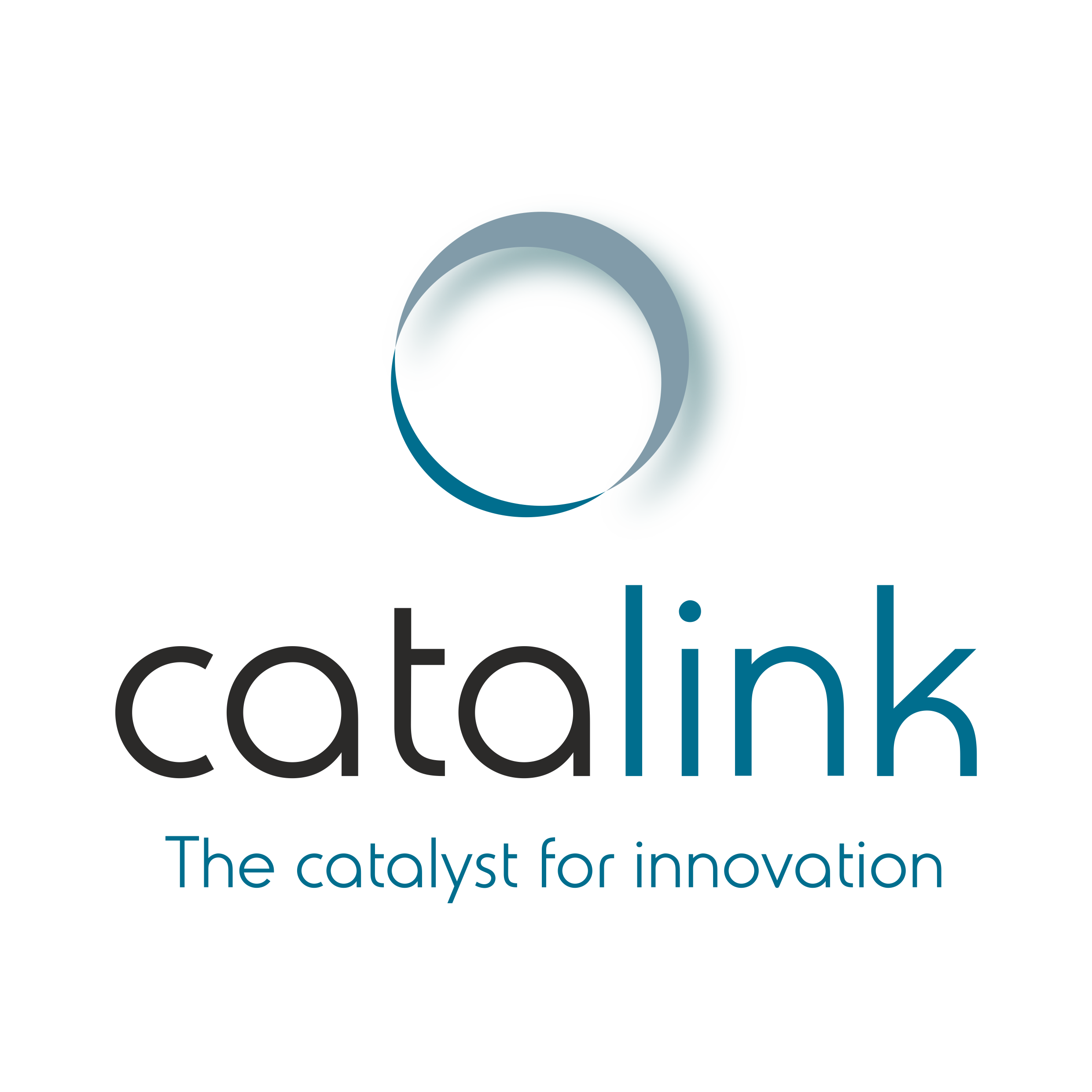
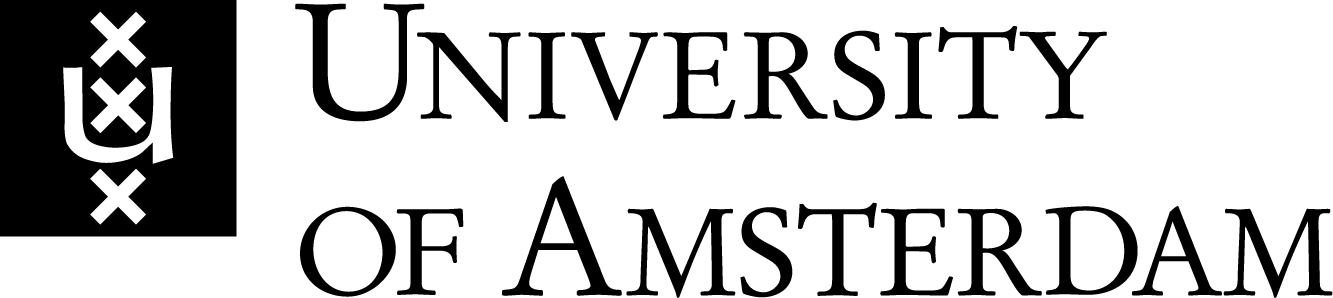
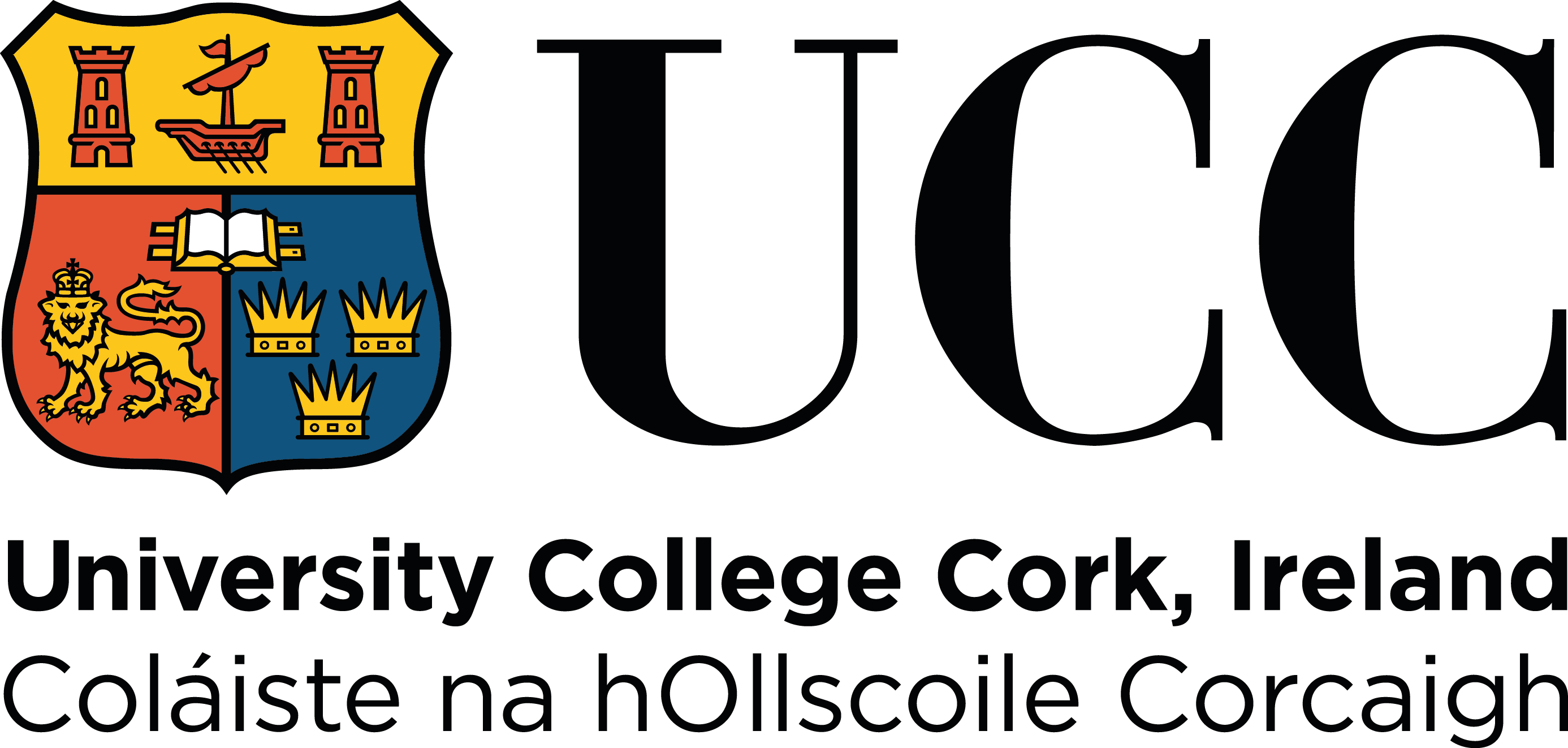
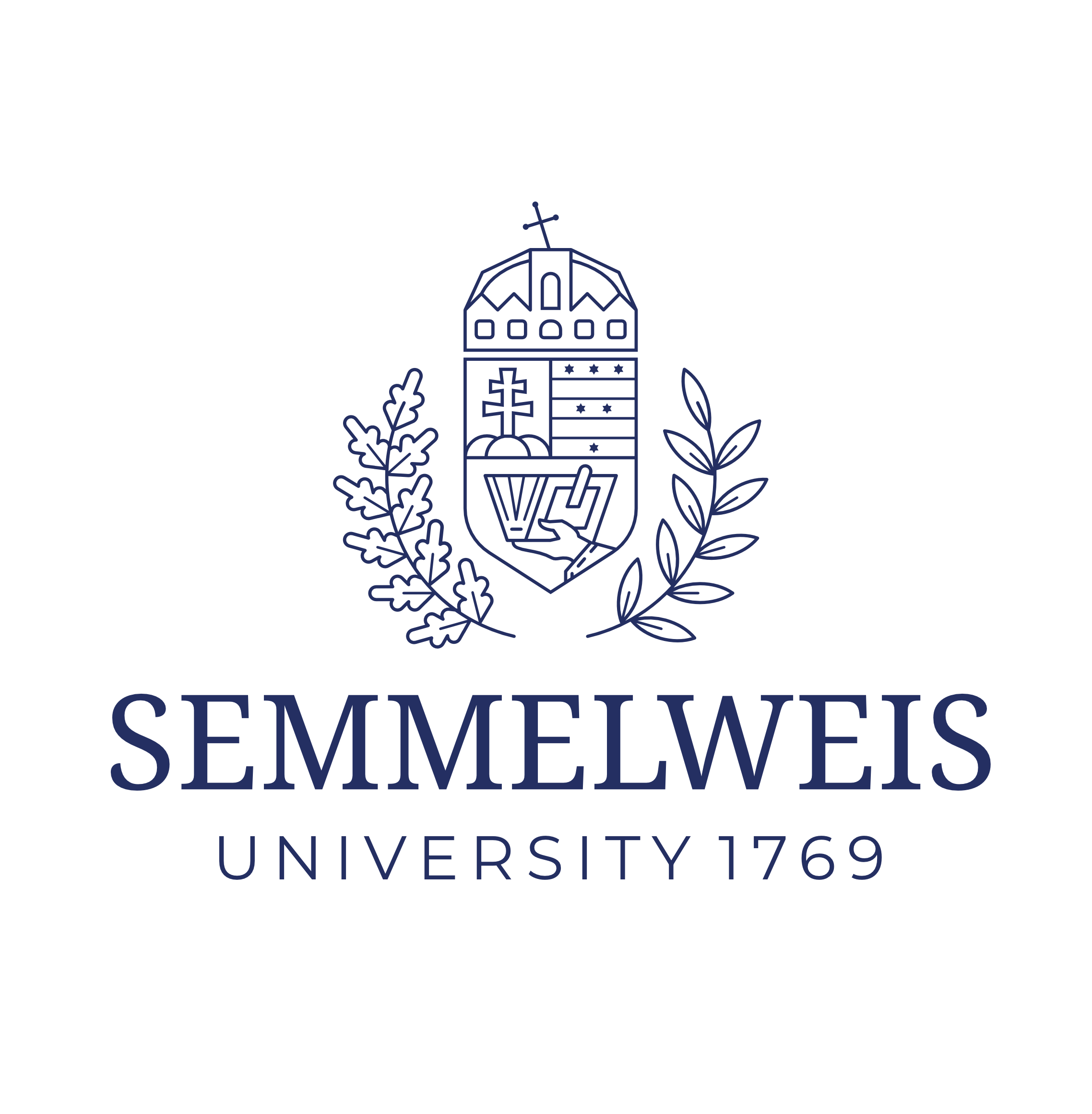
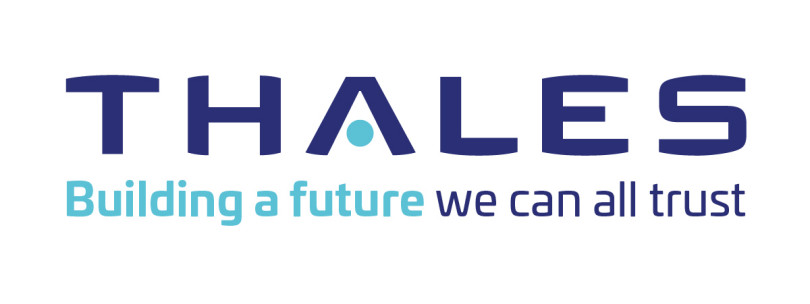
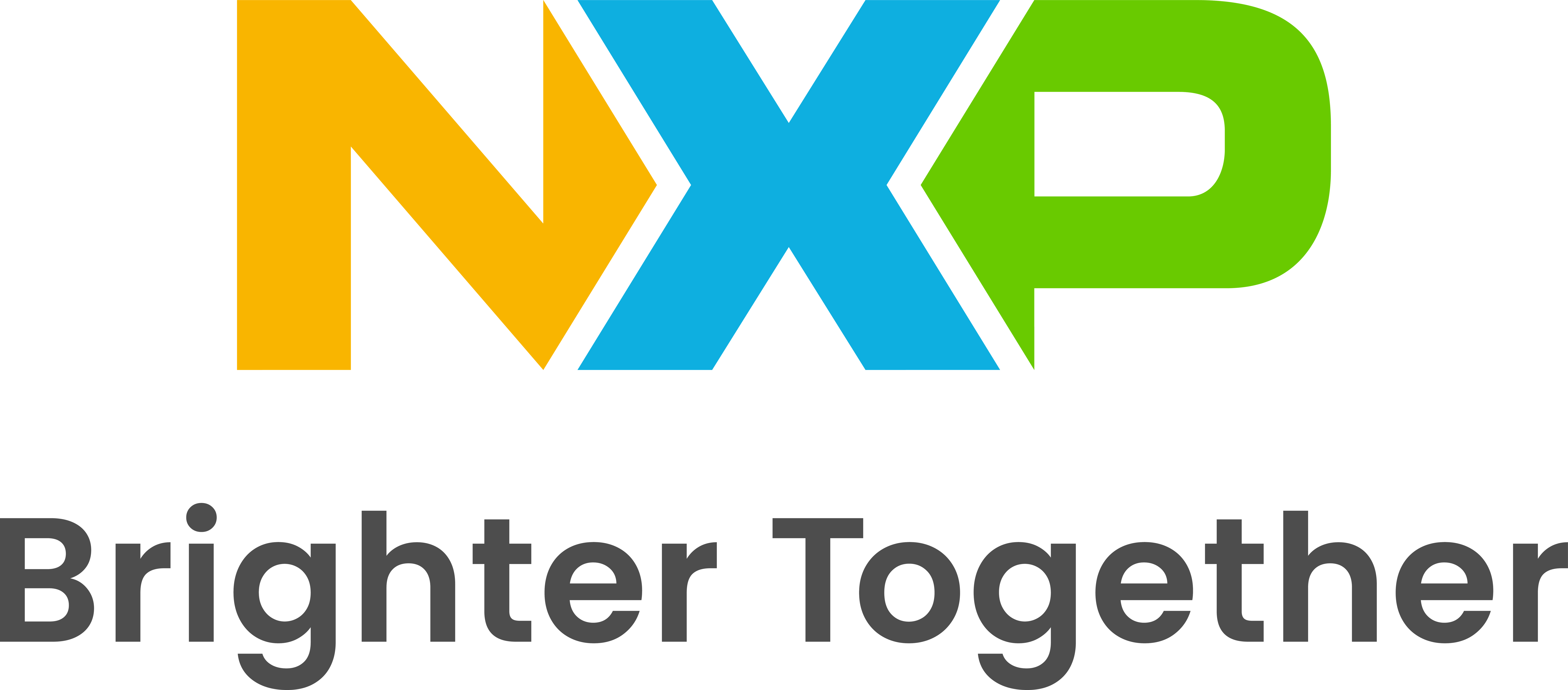
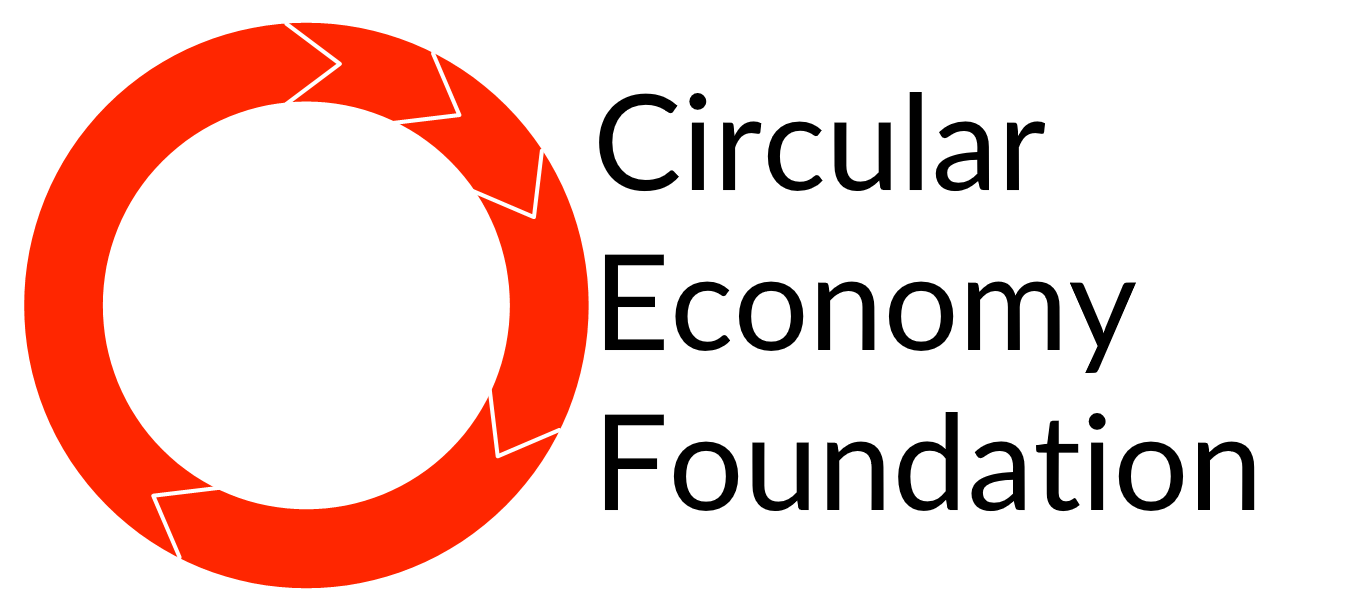
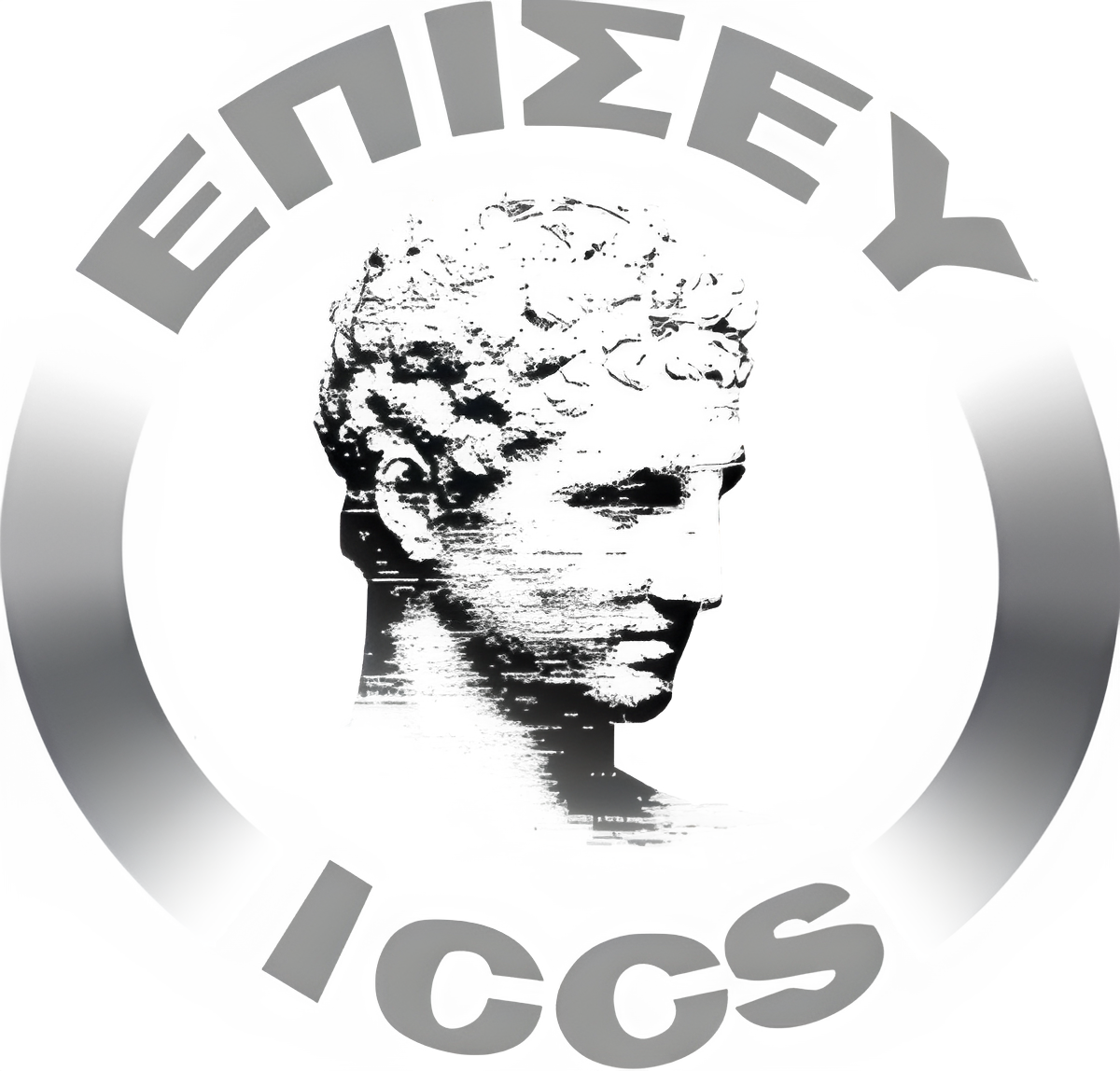
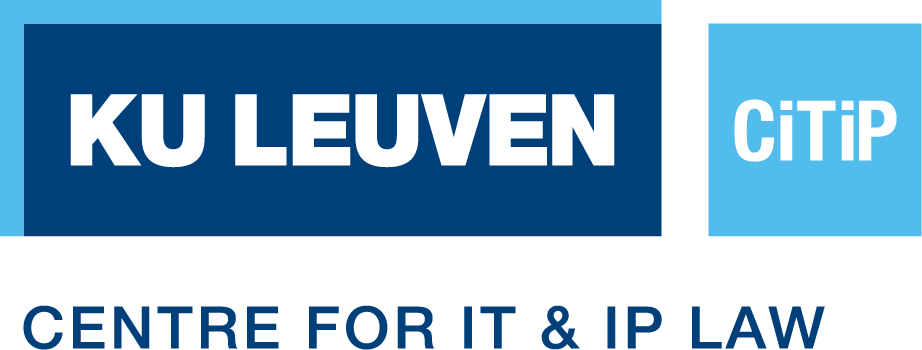
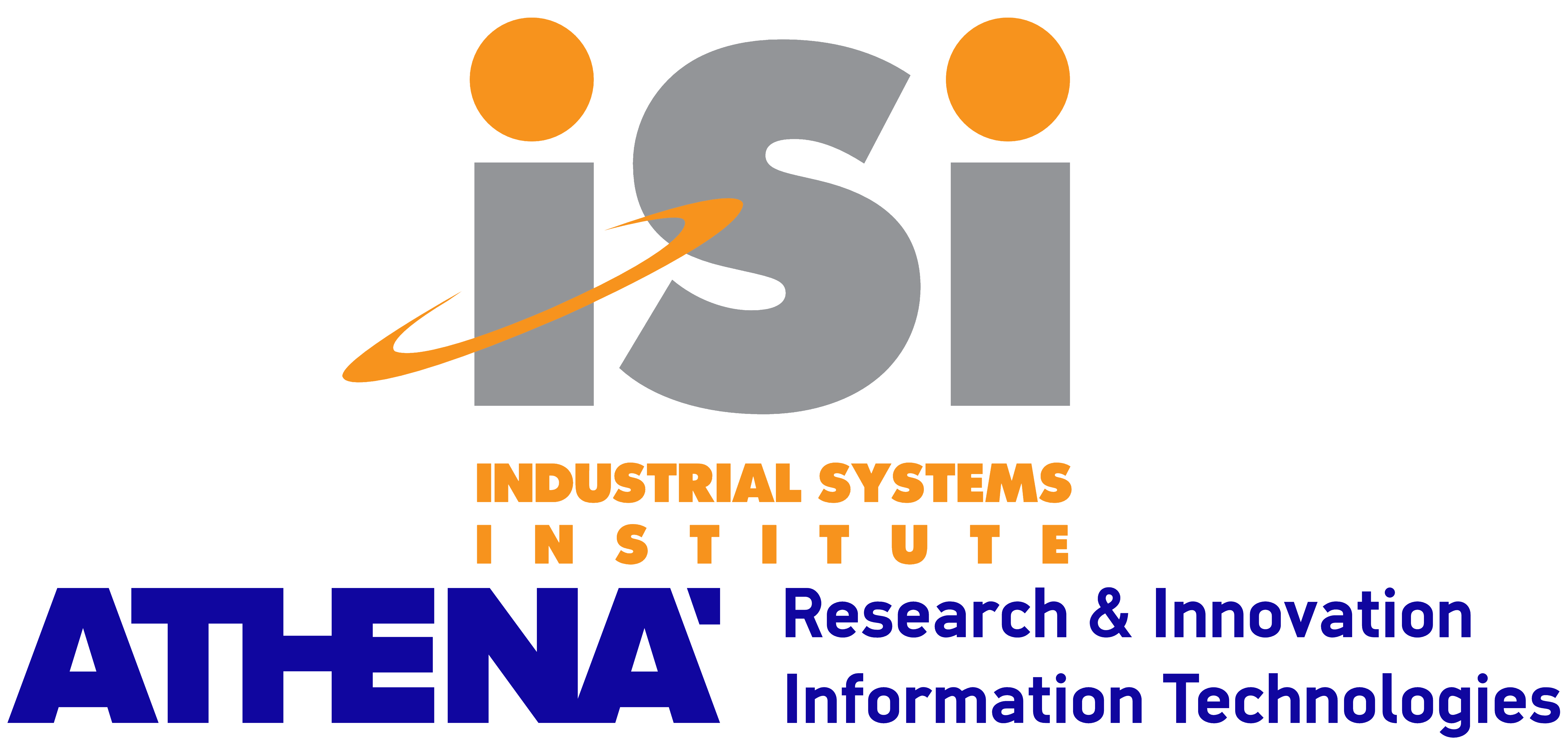

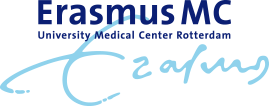
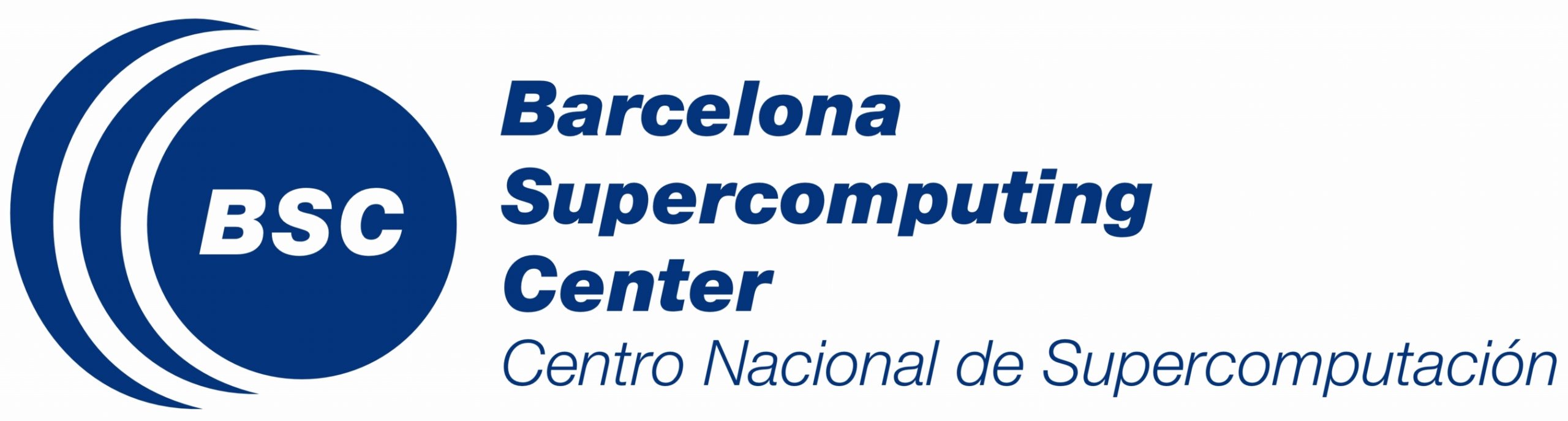
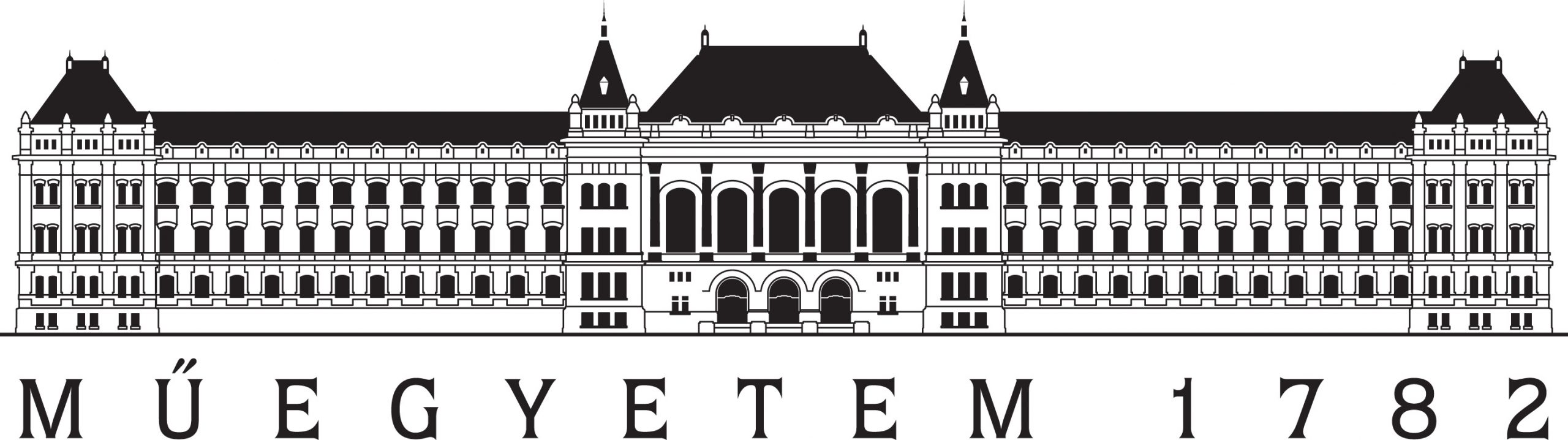
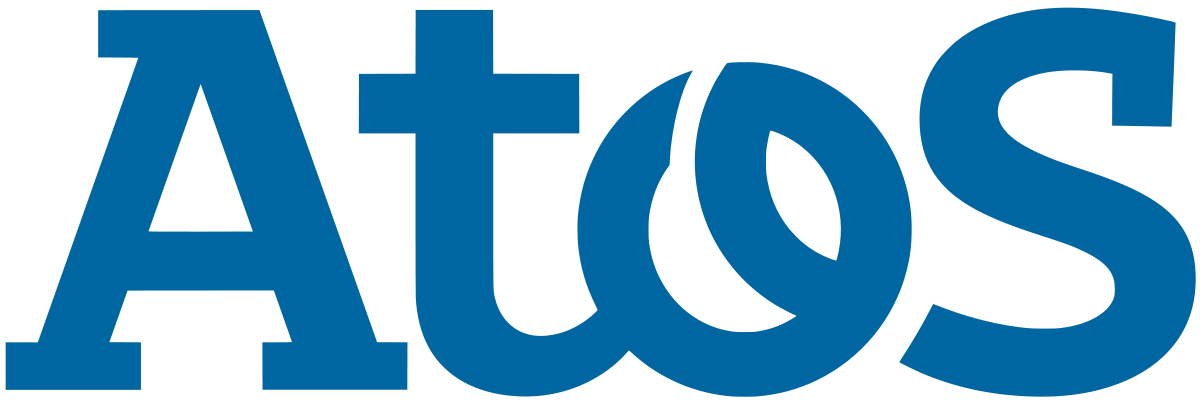